PhD Research Award of the European Physical Society for IPP scientists
Dr. Andrea Pavone awarded for his doctoral thesis on fast data analysis
Dr Andrea Pavone from Max Planck Institute for Plasma Physics in Greifswald has been awarded the 2021 Plasma Physics PhD Research Award of the European Physical Society (EPS) for his doctoral thesis “Machine learning approximation of Bayesian inference in nuclear fusion”. In recognition of outstanding research achievements, the EPS Plasma Physics Division awards up to four prizes annually to young scientists from all over Europe.
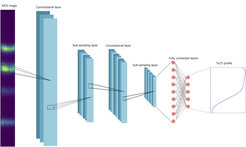
A measured X-ray image of the plasma emission (on the left) is fed as an input to an artificial deep neural network. The layers of the network (centre) process the input until the corresponding electron and ion temperature profile is found and output by the network (on the right).
In his work in the IPP division “Stellarator Heating and Optimisation”, Andrea Pavone dealt with data analysis in fusion experiments. In order to describe the complex plasma behaviour, many different measurements have to be evaluated and combined with each other. He therefore looked for a way to use state-of-the-art analysis methods but avoid excessive demand on computing capacity, and found a completely new approach: he accelerated the so-called Bayesian data analysis with the help of neural networks. This could significantly increase the applicability of the method.
Andrea Pavone began by determining the temperature profiles in the plasma of the Wendelstein 7-X fusion device: here, the temperatures of the ions and electrons of the plasma are determined, among other things, using the X-ray light emitted by heavy ions such as argon. The emission is observed along many lines of sight by detectors. The ion temperature can be determined from the broadening of the emission lines, and the electron temperature from the line ratios.
The Bayesian analysis method uses forward models: it starts from the initially unknown plasma parameters, which are varied – in a computationally very complex way – until the physical processes based on them reproduce the measurement result. In the given case, the atomic processes of the X-ray emission, their summation along the lines of sight and the many functional details of the X-ray detector had to be taken into account. To find a single set of profiles for the ion and electron temperatures in this way, a powerful workstation needs about half an hour. The detector, however, delivers data every ten milliseconds for many minutes – which makes the Bayesian method extremely time-consuming.
Here, Andrea Pavone’s approach comes into play. He succeeded in reducing the time required from 30 minutes to milliseconds: a neural network took over the computing task. The forward model alone is sufficient to train the network; measurement data are not required. While the forward model was tested on real data, Andrea Pavone was able to show that synthetic data are sufficient for training the neural network.
In further steps, he transferred the method to another fusion device – JET in Great Britain –and to another measuring method, namely the determination of the electron density by lithium beam spectroscopy. In doing so, he was not only able to considerably accelerate the analysis, but also improve the significance of the measurement results.
His approach of using synthetic data generated by the forward model for training the neural network could make automation of the analysis method possible. This would be very interesting for future devices such as ITER, but – far beyond fusion research – also for the study of any other complex physics system. The method of Bayesian analysis could thus find much broader application than has been possible so far because of the enormous computational effort involved.
The doctoral prize of 1000 euros will be awarded to Andrea Pavone at the upcoming Plasma Physics Conference of the European Physical Society, which will take place online from 21 to 25 June. All award winners will present their work there in a lecture.